The woman had noticed a worrying lump in her thyroid that didn’t go away. Her doctor ordered an ultrasound exam, and the results were concerning enough that a biopsy was done to see if the lump was cancerous.
The patient sought a second opinion from a radiologist who does thyroid ultrasound exams using artificial intelligence (AI), which provides a more detailed image and analysis than a traditional ultrasound. Based on that exam, the radiologist concluded with confidence that the tissue was benign, not cancerous — the same conclusion reached by the pathologist who studied her biopsy tissue.
“I’m an example of a patient who had a biopsy that could have been prevented by using AI,” says the patient, who had suffered weeks of anxiety and lost sleep while awaiting the final results from her doctor.
While AI is not commonly used in cancer diagnoses, more and more doctors are deploying it to help them determine what might be cancer, predict what might develop into cancer, and devise personalized treatment plans when cancer is found. By using AI to analyze images — including mammograms, sonograms, x-rays, MRIs, and tissue slides — doctors are getting more precise pictures, along with deeper analyses of what they see.
“It’s another tool to help us realize the promise of precision medicine,” says Tufia C. Haddad, MD, medical director of digital strategy at the Mayo Clinic Center for Digital Health in Minnesota, whose radiology and pathology colleagues at the clinic use AI for cancer diagnostics.
At a time when AI is increasingly utilized in health care systems for such processes as communication, data analysis, and administration, the technology is working its way into direct clinical care, especially in oncology. That’s largely because of its ability to analyze an image based on enormous amounts of data from thousands of images on which it is trained. The Food and Drug Administration has approved AI-assisted tools to help detect cancers of the brain, breast, lung, prostate, skin, and thyroid. Recent and ongoing studies show promising results for additional tools.
Radiologist Laurie Margolies, MD, director of breast imaging at Mount Sinai Health System in New York, likens her use of AI to consulting a brilliant colleague: “It would be like tapping someone on the shoulder and saying, ‘What do you think of this?’”
How it works
Diagnosing cancer from images and tissue samples is incredibly complicated and time-consuming. One reason is the limitless diversity of cancer cells. “Every cancer has unique elements,” says Olivier Elemento, PhD, director of the Englander Institute for Precision Medicine at Weill Cornell Medicine in New York. “Every piece of tissue that you get as a pathologist is unique in some ways. It has features you may not have seen before.”
The evaluation is a hands-on, labor-intensive process. For one patient, a pathologist might study numerous tissue slides and consider variables like the density, shape, and number of cells. When analyzing images, radiologists measure the size of tumors and draw outlines around them. When doctors go through synthesizing data and making a cancer treatment plan, “it might require five sub-specialists to analyze the information” and reach a conclusion supported by the evidence, says Alexander T. Pearson, MD, PhD, associate professor of medicine at the University of Chicago’s Division of Biological Sciences.
While many conclusions are straightforward, the assessment of images where the diagnosis is not so obvious can vary from doctor to doctor. Academic literature about improving the precision of cancer diagnoses refers to the problem of “interobserver variability” — different doctors reaching different conclusions from the same information.
This doesn’t mean cancer diagnoses are routinely wrong. It means that even with the use of sophisticated medical imagery devices that peer into the body, deciding what those images reveal remains an interpretive human task.
AI provides another interpretation. Trained on data from thousands of images and sometimes boosted with information from a patient’s medical record, AI tools can tap into a larger database of knowledge than any human can. AI can scan deeper into an image and pick up on properties and nuances among cells that the human eye cannot detect. When it comes time to highlight a lesion, the AI images are precisely marked — often using different colors to point out different levels of abnormalities such as extreme cell density, tissue calcification, and shape distortions.
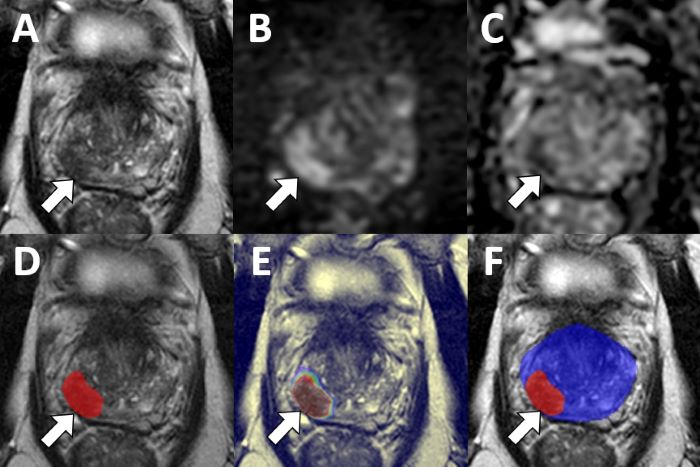
Standard MRI images (top row) of a patient’s prostate indicate a possible cancerous lesion. MRI images analyzed by an AI algorithm (bottom row) highlight the lesion with greater precision and with colors indicating the probability of cancer at various points. The physician diagnosed adenocarcinoma.
Translational AI group headed by Baris Turkbey, MD, National Cancer Institute
Benefits for doctors, patients
Doctors say the way AI-assisted imaging works can provide several important benefits for detecting and treating cancer. For example, Pearson describes what a pathologist sees in an AI-assisted analysis of a digitized biopsy slide from a thyroid, where “lesions of interest are very common.”
“What you would see is a highly magnified picture of the microscopic architecture of the tumor. Those images are high resolution, they’re gigapixel in size, so there’s a ton of information in them. The AI model recognizes patterns that represent cells and tissue types and the way those components interact,” better enabling the pathologist to assess the cancer risk.
The AI “doesn’t say there’s no chance of this being cancer,” Margolies says of an AI-assisted mammogram. “It says less than 1 in 2,500 mammograms we see [with these properties] are going to have breast cancer. Or, in some cases, 1 out of 6 of these mammograms show cancer in a spot that we marked.”
Depending on such results, she says, a doctor might order additional imaging or a biopsy because the risk appears to be high, or have a patient return for scans within six months. Other patients might continue with traditional screening because the risk appears to be low. Those decisions illustrate some of the direct benefits that AI-assisted imaging provides for patients.
First, by confidently diagnosing cancers sooner, doctors can move patients into treatment before the cancer spreads further. “The goal is that we find those cancers earlier than we would have otherwise,” Margolies says. “A cancer that maybe the naked eye would not have [detected] or that the overworked radiologist might not have spotted.”
Second, the AI tools can help assess what course of treatment might be most effective, based on the characteristics of the cancer and data from the patient’s medical history, Haddad says.
When the diagnosis is negative for cancer, AI tools can help avoid unnecessary follow-up biopsies. AI-assisted analyses help to reduce false positive diagnoses, says radiologist Ismail Baris Turkbey, MD, head of the Artificial Intelligence Resource Initiative at the National Cancer Institute in Maryland.
“A false positive puts an extra burden on the medical system and on the patient” in terms of time, cost, and invasive procedures, Turkbey says.
Speed is another benefit. Pearson notes that an assay of tissue using a traditional chemical stain on a slide might take days; AI-assisted analysis “on a piece of tissue is nearly instantaneous. It may take minutes to scan and seconds to analyze.”
The AI tools accelerate the assessment process in another way, Margolies says: By identifying cases that show a high risk of cancer, the tools alert radiologists to images that they might want to move up in their queue to examine.
“The patients the AI marked as a high concern for breast cancer can go to the front of the line,” she says.
Elemento, at Weill Cornell Medicine, hopes that AI tools will free up oncologists, radiologists, and pathologists “to focus more and more on the really complex, challenging cases” that require their reasoning skills and expertise.
Limits and learnings
Despite these advances, doctors acknowledge that AI is still in its infancy as a diagnostic tool.
Like most AI technology, the tools used for medicine have been found to sometimes produce hallucinations — false or even fabricated information and images based on a misunderstanding of what it is looking at. That problem might be partly addressed as the tools continue to learn; that is, as they take in more data and images, and revise their algorithms to improve the accuracy of their analyses.
Less clear is how those algorithms make their assessments — something that those who use and study AI call the “black box” problem.
“When there’s unsupervised learning [by the algorithm], you can’t necessarily see a formula or show how the computer came to this output,” Haddad says. “When the methods of combining the data or variables aren’t clear with an AI model, that raises the question, ‘How do you trust that?’”
Another question is whether biases get introduced into the algorithm. Pearson notes that questions could be raised about whether an AI model uses information from medical records to affect results based on the hospital where a biopsy was performed or a patient’s economic status.
On the other hand, Pearson says, AI tools might allow more deployment of fast and accurate oncology imaging into communities — such as rural and low-income areas — that don’t have many specialists to read and analyze scans and biopsies. Pearson hopes that the images can be read by AI tools in those communities, with the results sent electronically to radiologists and pathologists elsewhere for analysis.
For Haddad, the ultimate value of AI-assisted cancer diagnoses lies in health outcomes for patients: “The million-dollar question will be, ‘Are we catching things early enough so that we can intervene and change the trajectory of a disease or complication from a disease?’”
Hadded hopes that as AI tools take in and use more data, they will improve the ability of doctors to get ahead of the curve on a patient’s changing health conditions and which treatments might work best for them. “I think that AI is going to help usher in the era of medicine that is more proactive than reactive.”